Optimizing the AI Rush: Cast AI’s Funding Targets a Critical Enterprise Headache
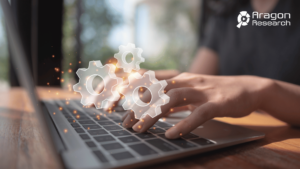
Optimizing the AI Rush: Cast AI’s Funding Targets a Critical Enterprise Headache
The rapid adoption and scaling of artificial intelligence initiatives, from model training to inference, have created unprecedented demands on computing resources. This surge in activity, while driving innovation, has quickly translated into significant cost and resource management challenges for organizations worldwide. Addressing this critical pain point, Cast AI, a startup focused on automating and optimizing workloads for AI and other tasks, recently announced a substantial funding round, signaling strong market validation for their approach.
This blog overviews Cast AI’s recent funding news and analyzes its focus on tackling the escalating costs of AI workloads.
Why the Focus on AI Workloads for Cast AI?
Cast AI has secured $108 million in Series C funding to advance its platform for optimizing cloud and on-premise workloads, with a particular emphasis on the demanding requirements of artificial intelligence. The company highlights that the intensive compute needs for training and running AI models are creating major cost and resource inefficiencies for enterprises. According to Cast AI CEO and co-founder Yuri Frayman, the core challenge lies in the efficient utilization of GPUs, compute power, and electricity.
Cast AI’s platform aims to automate the analysis of infrastructure capacity across various environments, including major cloud providers and on-premise setups, to identify the optimal cost-performance ratio for distributing workloads. The company cites internal research suggesting low average utilization rates for existing compute resources, including GPUs, indicating a significant opportunity for optimization. The new funding will support further research and development to enhance their automation capabilities specifically for AI, alongside expanding their market presence, particularly in the U.S.
Analysis
From an Aragon Research perspective, Cast AI’s significant funding round and explicit focus on AI workloads underscore a crucial and rapidly growing pain point for enterprises. The initial rush to leverage AI often overlooks the underlying infrastructure economics. As organizations scale their AI initiatives, the inefficient allocation and underutilization of expensive resources like GPUs can lead to ballooning cloud bills and strained IT budgets. Cast AI’s value proposition directly addresses this by automating the complex task of optimizing workload placement and scaling across hybrid and multi-cloud environments.
The reported low utilization rates (10% for CPUs, 23% for memory, and similar trends likely for GPUs before optimization) highlight a widespread inefficiency that many organizations struggle to manage manually. Automating the process of analyzing capacity, predicting needs, and dynamically adjusting resources for AI training and inference jobs can yield substantial cost savings and improve operational efficiency.
The “near unicorn” valuation achieved by Cast AI and their rapid customer acquisition rate (2,100 customers in three years, including major enterprises) provide strong evidence of the acute market need for solutions that can bring FinOps principles and automated optimization to the high-stakes world of AI infrastructure. This signals a market trend where optimizing the execution of AI workloads is becoming as critical as the AI model development itself.
What Should Enterprises Do About This News?
Enterprises that are currently undertaking or planning significant investments in AI development and deployment should view Cast AI’s funding news as a validation of the importance of AI workload optimization. If your organization is experiencing escalating costs related to AI compute, or if your infrastructure teams are struggling to efficiently manage GPU and other resources across different environments, you should actively investigate solutions like Cast AI.
Evaluate your current cloud and on-premise resource utilization for AI workloads. Understand the actual costs associated with training and running your models. Consider conducting a proof of concept with an AI workload optimization platform to assess its ability to deliver tangible cost savings and efficiency improvements. Don’t assume that simply acquiring powerful hardware or cloud instances is sufficient; optimizing how those resources are used is paramount to achieving a positive return on your AI investments.
Bottom Line
The substantial funding secured by Cast AI for its focus on AI workload optimization highlights a critical challenge facing enterprises today: the high cost and inefficiency of powering the AI revolution. As organizations scale their AI initiatives, automating the management and optimization of underlying compute resources, particularly expensive GPUs, is no longer a luxury but a necessity.
Cast AI’s growth indicates strong market demand for solutions that can deliver significant cost savings and improved efficiency in this domain. Enterprises heavily invested in AI should take this as a clear signal to prioritize the optimization of their AI infrastructure and actively evaluate platforms designed to address this complex and costly problem.
UPCOMING EVENT
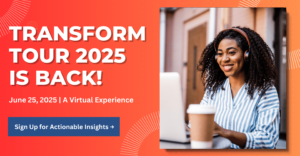
We invite you to join us for Aragon’s June Transform Tour, a virtual event designed to equip business leaders with actionable insights into driving real-world results through AI and strategic planning.
This event features two focused sessions:
Session 1: A Practical Guide to Strategy, Architecture, and Operations – Unlock Tangible Business Value from AI
Many organizations struggle to move beyond AI hype to real-world results. During this session, we will provide actionable insights into crafting a clear, business-driven AI strategy, architecture, and operations framework. We’ll explore how to establish effective governance, build the right organizational structures and Centers of Excellence, design robust AI architectures, develop practical roadmaps, and implement a proactive security strategy.
Join us to discover:
- How a proactive and practical AI strategy can significantly decrease risk.
- How to leverage your AI strategy to effectively guide architecture and governance decisions.
- Practical change management approaches to ensure successful and widespread AI adoption.
Equip yourself with the knowledge to translate AI’s promise into measurable business impact.
Session 2: A Practical Guide to Development, Training, Management and Security
Navigating the complexities of AI development, deployment, and security requires a solid technical foundation. The emergence of this new software and hardware technology stack requires mastering introducing new development, integration, data management and technology architecture skills. This webinar offers practical guidance for IT leaders on building efficient training datasets and pipelines, selecting the right development frameworks, implementing robust security measures across the AI lifecycle, and establishing effective management practices for your AI infrastructure.
We will address critical questions such as:
- How does AI fundamentally change the IT landscape?
- What are the best practices for developing and managing AI?
- How do IT leaders and developers support security, integration and data management?
Have a Comment on this?