The Challenge to Combat Circular Bias in AI Systems
The Challenge to Combat Circular Bias in AI Systems
Let’s say you are looking for a product on a website. You look at the stars rating and it shows 5 stars but there are a few ratings of 1 star. Would you buy the product? Probably because the positive consensus is strong. However many reviews happen early in the product purchase. The 1-star rating might have come from someone who has used the product for a longer term and had insights into cases where the product does not perform as expected.
When Artificial Intelligence (AI) systems are built, the above rating material and descriptions will become part of the training material and most certainly reinforce the positive aspects of the product. Then as bloggers and influencers offer product opinions, certainly in the future using AI to gather information, those reviews likely will skew further toward the positive which in turn will become future training material for the AI systems other product reviewers might use.
However, a competent journalist would likely mention the unfortunate experiences and qualify why such experiences should be given attention, namely because of the depth of use by the reviewer. The journalist might also offer implications of the negative review and why it might hold more weight than the sheer number of positive reviews.
Circular bias, where an AI system’s outputs reinforce the biases present in its training data, is a major challenge in AI development. Here are some ways technologists are working to combat it:
- Data Cleaning and Augmentation: Techniques like identifying and removing biased examples from training data, or enriching datasets with more diverse examples, can help reduce the influence of existing biases. This can be labor intensive even when automation is used.
- Fairness Metrics and Algorithms: Researchers are developing metrics to quantify bias in AI models and algorithms that can mitigate bias during training. This can involve weighting training examples or adjusting model architectures to be less sensitive to specific features. However, there are really few accepted methods to do this.
- Human-in-the-Loop Training: Integrating human oversight into the training process allows for real-time identification and correction of biased outputs. This can involve techniques like active learning, where the model identifies potentially biased decisions and queries a human for guidance. We believe this is the most active solution today, but the cost and time requirements can be exhaustive.
- Explainable AI (XAI): Developing methods to understand how AI models arrive at their decisions can help identify where biases might be creeping in. By understanding the reasoning behind an AI’s output, it becomes easier to pinpoint and address bias. Long term this holds promise but the technology is in its infancy.
- Promoting Transparency and Collaboration: Openly sharing datasets and algorithms allows for broader scrutiny and identification of potential biases. Collaboration between researchers, developers, and ethicists is crucial for developing robust methods to combat bias. This is a policy solution that is helpful but likely will not result in an end result deemed to have mitigated circular bias.
Related Article: 4 AI Essential Rules: Prompt, Review, Verify, Repeat
Bottom Line
Combating circular bias is an ongoing area of research, but these techniques offer promising avenues for building fairer and more reliable AI systems.
However, combatting circular bias will largely be relegated to the user to closely examine AI output relative to the task input to the algorithm. Web searches to assess the raw data can be useful to rationalize the AI output against human common sense, although the reviewers themselves must guard against personal bias. Team reviews may be the best choice before any such AI output is put into production and dissemination.
UPCOMING WEBINAR
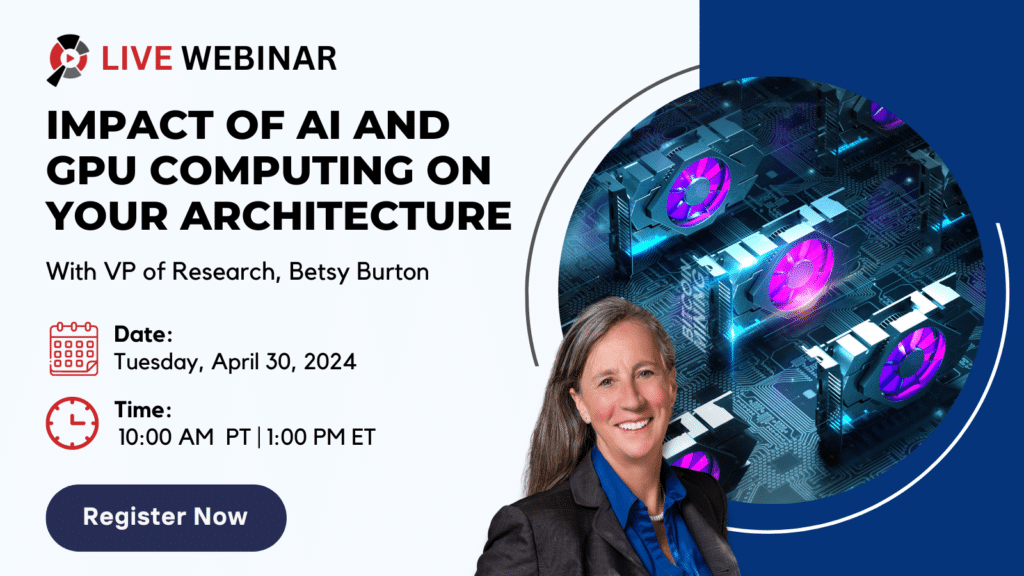
Impact of Artificial Intelligence & GPU Computing on Your Architecture
The move toward cloud computing has been underway for years, but nearly all of the profits have gone to a handful of vendors. Artificial intelligence and GPU computing models are beginning to change this trend.
The era of edge computing era is here and with it will come new deployment and business models.
Key topics to be covered in this webinar:
- Artificial intelligence and Computing Trends Driving Modern Architectures
- Architecture Scenario for 2026
- Prepare for these New Architecture Models
Have a Comment on this?